Nasim Abdollahi, Medical Biophysics
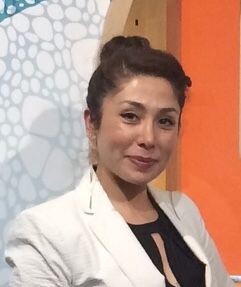
Supervisor:
Dr. Bo Wang, Assistant Professor, Temerty Faculty of Medicine, University of Toronto, Lead Artificial Intelligence Scientist, Peter Munk Cardiac Centre and the Techna Institute at the University Health Network (UHN), CIFAR AI Chair, Faculty Member at Vector Institute
PhD Thesis:
Microwave imaging algorithms: Enhancement and new approaches
PhD Description:
MWI algorithms create quantitative images of the dielectric properties of the object of interest (OI) from measured scattered electromagnetic fields obtained by interrogating the OI with non-ionizing and low-power microwave radiation in a non-invasive procedure. Developing MWI algorithms is challenging because of the non-linearity and ill-posedness of MWI inverse problems. The ill-posedness is a fundamental complication influenced by insufficient amount of information about the OI, which is limited to the measurements. State of-the-art MWI algorithms solve the nonlinear electromagnetic inverse problems by means of iterative optimization methods that are computationally intensive procedures. The ill-posedness is addressed by means of applying regularization techniques. This thesis focused on investigating and developing MWI techniques in an attempt to mitigate these challenges and improve the accuracy and resolution of the MWI reconstructions. First, a novel non-iterative algorithm, which is formulated based on the eigenfunction basis of the Helmholtz operator and referred to as Non-Iterative Eigenfunction-based Inversion (NIEI), was introduced. The formulation of the NIEI algorithm implements a novel regularization approach that exploits the fact that contrast source is zero outside the imaging domain and incorporating this information in form of constraints at virtual points or contours. Unlike the conventional imaging algorithms, the NIEI algorithm does not require standard discretization strategies and the unknowns are represented by expanding in terms of whole-domain eigenfunction basis. Hence, the inverse problem becomes recovering the coefficients of unknown expansions rather than finding the dielectric property of discrete elements. Therefore, the number of unknowns in the inverse problem is reduced significantly and this itself contributes to regularizing the problem to some degree. The NIEI system matrices are well-posed and the inverse source and scattering problems are solved by direct matrix inversion. The performance of the NIEI algorithm is studied using several synthetic test problems. The results compare very well with state-of-the art inversion algorithms, while being computationally more efficient and cutting-edge form WI inverse problems. Second, the choice of proper constraints from various options for regularizing the NIEI inverse source solver is investigated and assessed on synthetically obtained forward data. It is shown that the proper selection of constraint equations can significantly enhance the performance of the NIEI algorithm. Additionally, a multi-modality technique that uses ultrasonic structural information as prior information for quantitative microwave breast imaging is explored. The use of prior information provides regularization and improves the stability of the inversion algorithm. The structural information is incorporated as an inhomogeneous numerical background into a state-of-the-art finite-element method contrast source inversion (FEM-CSI) algorithm. The multi-modality approach is assessed using synthetic data obtained from several MRI-derived anthropomorphic breast models. Significant improvement in recovering interior structure of the breast and detecting the tumors is observed compared with previous studies on using radar-based structural prior information. Moreover, a multistage microwave breast imaging algorithm is proposed that incorporates the low-resolution eigenfunction-based reconstruction as prior information into the FEM-CSI technique in an approach that is similar to incorporating the ultrasonic prior information. However, this algorithm is a single-modality imaging strategy that deploys only electromagnetic field data obtained from a single microwave set-up. The algorithm efficacy is assessed by performing numerical experiments on anthropomorphic breast models at different frequencies. Reconstructed images confirm that incorporating the eigenfunction-based reconstruction as prior information improves quantitative reconstructions of the breast interior compared with the imaging results of previously reported multi-physics techniques. It is also shown that the approach is robust to different levels of resolutions of the utilized eigenfunction-based prior information. Finally, a 3D extension of the NIEI formulation is proposed. The non-iterative formulation offers a unique strategy based on the magnetic vector potential, where three scalar components of the contrast source vector are reconstructed from three direct matrix inversions (Stage-I). This is followed by another direct matrix inversion for reconstructing 3D profiles of complex contrast and permittivity (Stage-II).
Current Research:
In collaboration with Cyclica Inc. and Vector Institute, Dr. Abdollahi is working on developing NodeCoder, a unified framework based on graph convolutional network, that uses structure-augmented protein representations from the AlphaFold2 database to rapidly model any residue-based classification. Recent advances in protein structure predictions by AlphaFold2 and RoseTTAFold have unlocked the dark proteome, structurally-enabling thousands of new proteins to biotechnology and pharmaceutical applications. However, predicted protein structures lack the context that experimental studies provide to interpret protein functions, such as binding sites and macromolecular interfaces. NodeCoder generates graphs describing the 3D structure of each protein, where nodes correspond to individual amino acid residues and edges represent inter-residue contacts within a pre-set distance. The generated graphs and node features extracted from protein structure are then used for training the NodeCoder’s graph-based models. Trained models annotate specific regions of interest based on the structural attributes of the residues and their local neighbors. For instance, post-translational modifications and ligand binding sites of proteins are directly modeled without the need for experimental structure coverage in the PDB. The capability of the NodeCoder framework for residue characterization is assessed across different tasks, where the performance curves are representative of a high-quality residue characterization model.
Scholarship and/or Awards
- Mitacs Accelerate Postdoctoral Internship (2021)
- Mitacs Accelerate PhD Internship (2020)
- University of Manitoba Emerging Leader Award (2017)
- University of Manitoba Graduate Fellowship (2016)
- University of Manitoba International Graduate Student Scholarship (2016)
- University of Manitoba International Graduate Student Entrance Scholarship (2015)
- IEEE Women in Engineering International Leadership Conference Travel Award (2017)
Publications
- “Residue characterization on AlphaFold2 protein structures using graph neural networks”, Machine Learning for Structural Biology Workshop, NeurIPS 2021
- “Evaluating Performance of Microwave Image Reconstruction Algorithms: Extracting Tissue Types with Segmentation Using Machine Learning”, MDPI Journal of Imaging, 2021
- “MWSegEval—An image analysis toolbox for microwave breast images”, Elsevier SoftwareX, 2021
- “Improved Tumor Detection via Quantitative Microwave Breast Imaging Using Eigenfunction-based Prior”, IEEE Transactions on Computational Imaging, 2020
- “Incorporation of Ultrasonic Prior Information for Improving Quantitative Microwave Imaging of Breast”, IEEE Journal on Multiscale and Multiphysics Computational Techniques, 2019
- “Non-Iterative Eigenfunction-based Inversion (NIEI) Algorithm for 2D Helmholtz Equation”, Progress In Electromagnetics Research - PIERB, 2019
Visit Google scholar for complete list of publications.